Health Analytics | AI Diagnostics
Turning terabytes of clinical data into sub‑second, life‑saving insights—HIPAA‑secure, FDA‑ready
The global market for AI‑driven diagnostics is already $1.59 billion (2024) and growing 22.4 % CAGR (Grand View Research). Yet diagnostic errors still cost the U.S. system $100 billion+ a year and harm hundreds of thousands of patients (NCBI). Studies show AI can boost cancer‑detection rates 20 % when paired with clinicians (Health), and the FDA has now cleared 1,016 AI/ML medical devices (U.S. Food and Drug Administration), signaling mainstream momentum—if health systems can deploy safely and at scale.
Our promise: VarenyaZ fuses real‑time data platforms, explainable ML, and rigorous validation so your care teams diagnose faster, miss less, and stay fully compliant.
The AI‑Diagnostics Pressure Cooker
VarenyaZ Value Playbook
Unlock the full power of your clinical data with six core capabilities.
Unified Imaging & Lab Lake
DICOMweb + FHIR stream to Snowflake in < 1 min
Explainable ML Pipelines
SHAP & Grad‑CAM overlays for radiologists, CAP/CLIA tracebacks
Continuous Validation
MLOps with FDA SaMD pre‑checklists, locked‑weight snapshots
Edge & Cloud Inference
ONNX + NVIDIA Triton run CT in < 200 ms p95
Bias & Drift Sentinel
Feature drift, demographic parity, automated retrain triggers
Compliance by Design
HITRUST, HIPAA, GDPR, SOC 2 Type II—OPA policies & audit API
Modular Solution Stack
Composable architecture from data ingestion to explainable AI inference.
Capability
DICOM/PACS, HL7, FHIR, device streams
Core Tech
Orthanc, DICOMweb, Debezium, Kafka
Capability
Real‑time + batch, lineage, TTL
Core Tech
Feast v3, Snowflake, Redis Vector
Capability
Vision, NLP, multimodal
Core Tech
PyTorch 2, MONAI, Hugging Face Transformers
Capability
GPU batch, CPU edge, auto‑scale
Core Tech
NVIDIA Triton, ONNX Runtime, KServe
Capability
SHAP, Grad‑CAM, Lime, What‑If
Core Tech
Captum, IBM AIX360
Capability
SMART on FHIR apps, PACS overlays
Core Tech
React + MUI, Epic Hyperdrive
Capability
Drift, latency, ROC, recall
Core Tech
Evidently AI, Grafana Cloud
Capability
FDA SaMD trace, PHI masking
Core Tech
MLflow Registry, Vault, OPA Rego
Segment‑Specific Expertise
Pre‑built workflows, AI models, and compliance for each specialty—launch faster and safer.
Radiology & Imaging
- •Triage flags, zero‑click CAD, auto‑measurements
Pathology
- •WSI slide QC, mitosis detection, molecular stain prediction
Cardiology
- •ECG arrhythmia ML, echo strain analytics, FHIR waveform ingest
Ophthalmology
- •DR grading, OCT segmentation, mobile fundus AI
Population Health
- •Predictive risk, gaps‑in‑care stratification, SDOH fusion
Accelerator kits trim validation & deployment time 40–60 % per specialty.
AI‑Diagnostics Maturity Curve
From pilot to autonomous AI, each step powered by robust data & compliance.
KPI Ceiling
One model, single site
Blockers
Data silos
VarenyaZ Accelerator
FHIR Lake Ingest in 6 wks
KPI Ceiling
PACS overlay
Blockers
Workflow lag
VarenyaZ Accelerator
Edge Inference + Smart Cache
KPI Ceiling
FDA 510(k) clearance
Blockers
Doc bundles, traceability
VarenyaZ Accelerator
SaMD Validation Toolkit
KPI Ceiling
Auto‑retrain on new data
Blockers
Drift, bias
VarenyaZ Accelerator
Bias‑Sentinel & CI CD4ML
KPI Ceiling
Full multimodal AI MDT
Blockers
Culture
VarenyaZ Accelerator
Human‑in‑the‑Loop UX & guardrails
Proven Impact
Median across three 2024 hospital networks.
Stroke CT‑to‑Report
Cancer Detection (PPV)
Missed Findings / 1 k
Clinician Clicks/Study
Cost per Read
Signature Case Story — Oncology Network
AI for lung‑nodule CT flagged subtle lesions invisible in 2 D slices.
Results:
- p95 inference 140 ms
- Stage 1 detections + 22 %
- Radiologist TAT – 58 %
- 510(k) clearance in 7 months
Partner Ecosystem
Proven AI, cloud, and PACS/EHR integrations for frictionless deployments.
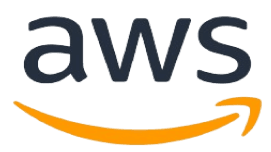
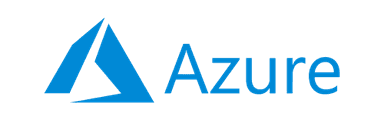
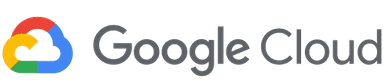
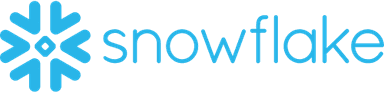

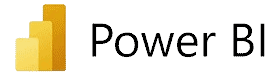
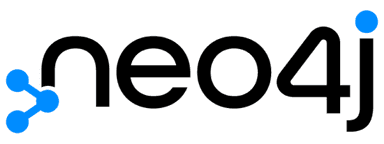
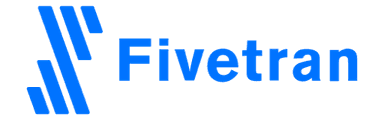
Ready to Diagnose Faster, Safer, Smarter?
Book a 30‑minute AI‑diagnostics consult — receive a latency audit, validation roadmap, and ROI model, free.
VarenyaZ — data to diagnosis, without delays or doubt.
Frequently Asked Questions
Everything you need to know — or just ask us directly.
How long to deploy AI CT triage?
What is the FDA path?
Can we validate without PHI leakage?
GPU cost concerns?
Explainability for radiologists?
How do you handle bias?
What about HL7 V2 labs?
Edge vs. cloud inference?
Can we plug in third‑party models?
Data residency for EU centers?
Does XAI slow inference?
Do you support non‑imaging AI (ECG, EEG)?
How is clinician feedback looped?
What uptime can we guarantee?
Who owns models & code?